在處理大量數(shù)據(jù)時(shí),有必要將具有特征的空間壓縮為向量。一個(gè)例子是文本嵌入,它是幾乎所有 NLP 模型創(chuàng)建過程中不可或缺的一部分。不幸的是,使用神經(jīng)網(wǎng)絡(luò)處理這種類型的數(shù)據(jù)遠(yuǎn)非總是可能的——例如,原因可能是擬合或推理率低。
下面是我提出一種有趣的方法來使用,這個(gè)方法就是很少有人知道的梯度提升。
數(shù)據(jù)資料
在最近一項(xiàng)有關(guān)于卡格爾的比賽結(jié)束了,在那里展示了一個(gè)包含文本數(shù)據(jù)的小數(shù)據(jù)集。我決定將這些數(shù)據(jù)用于實(shí)驗(yàn),因?yàn)楸荣惐砻鲾?shù)據(jù)集標(biāo)記得很好,而且我沒有遇到任何令人不快的意外。
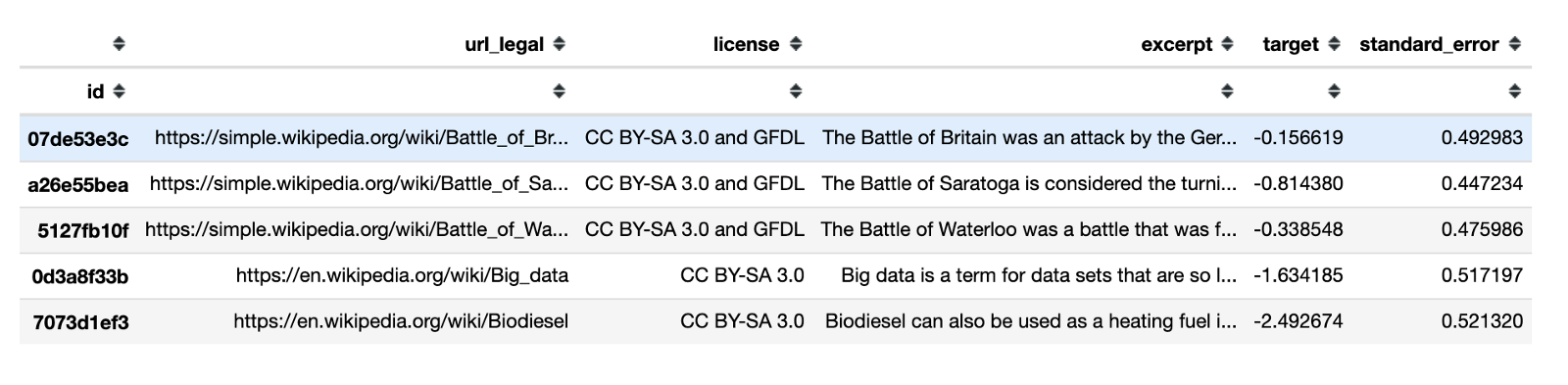
列:
- id - 摘錄的唯一 ID
- url_legal - 來源網(wǎng)址
- license - 源材料許可
- excerpt - 預(yù)測閱讀難易度的文本
- target - 更容易理解
- standard_error -測量每個(gè)摘錄的多個(gè)評分員之間的分?jǐn)?shù)分布
作為數(shù)據(jù)集中的目標(biāo),它是一個(gè)數(shù)值變量,提出解決回歸問題。但是,我決定用分類問題代替它。主要原因是我將使用的庫不支持在回歸問題中處理文本和嵌入。我希望開發(fā)者在未來能夠消除這個(gè)不足。但無論如何,回歸和分類的問題是密切相關(guān)的,對于分析來說,解決哪個(gè)問題沒有區(qū)別。
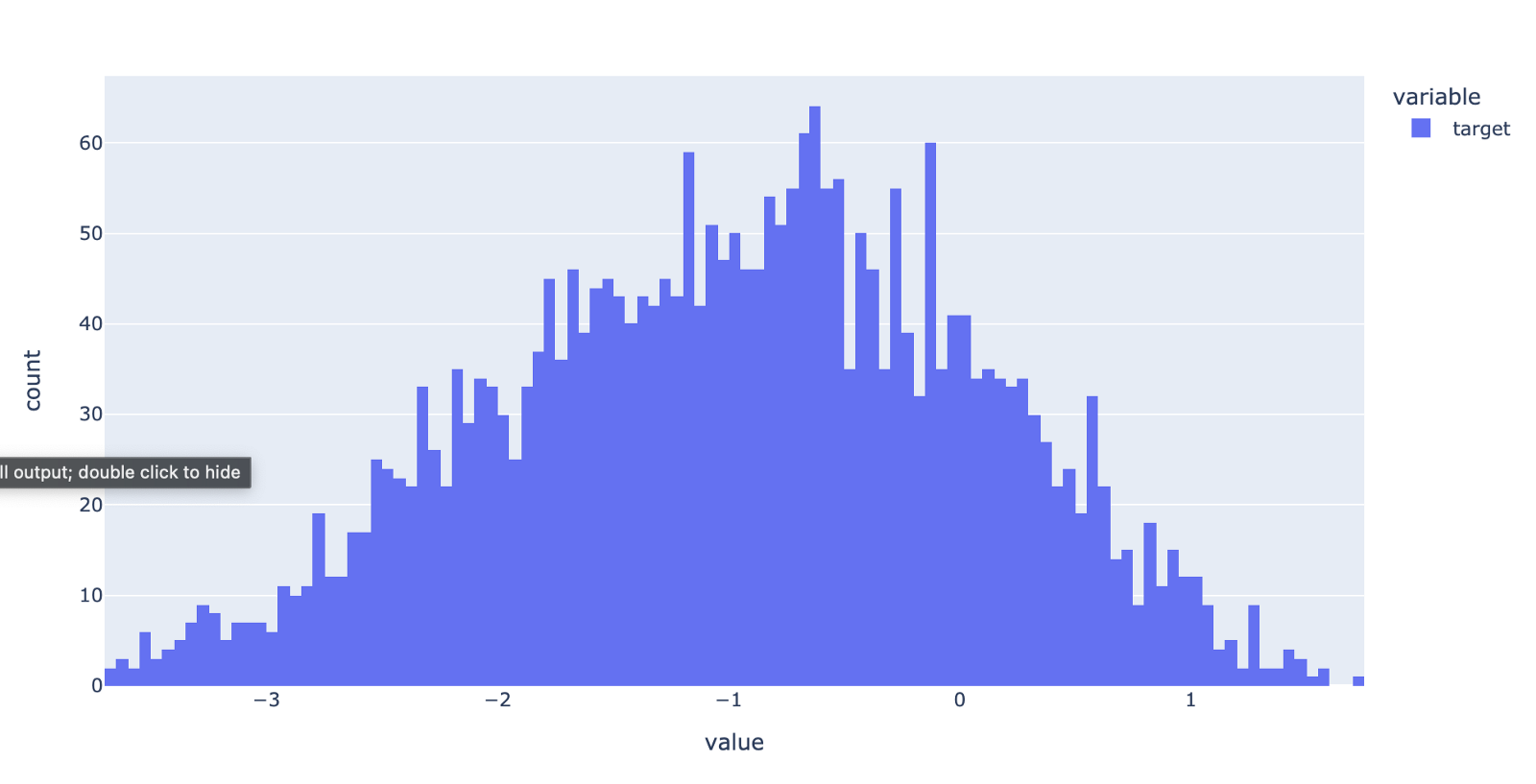
讓我們通過 Sturge 規(guī)則計(jì)算 bin 的數(shù)量:
num_bins = int(np.floor(1 + np.log2(len(train))))
train['target_q'], bin_edges = pd.qcut(train['target'],
q=num_bins, labels=False, retbins=True, precision=0)
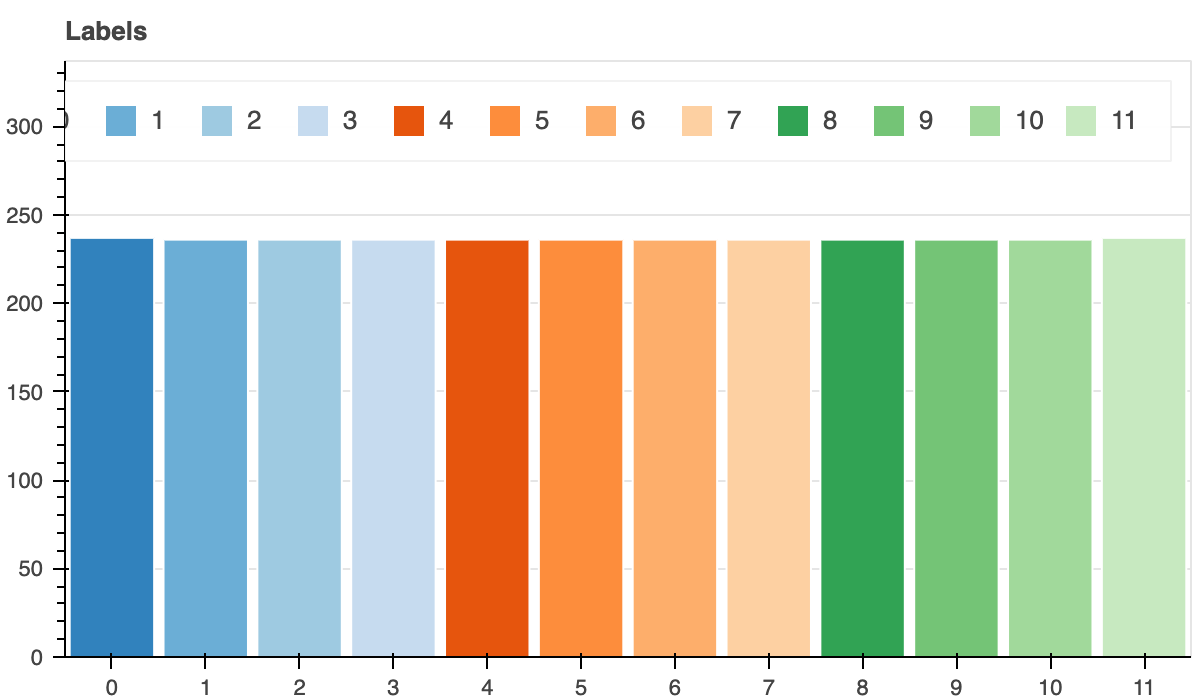
但是,首先,我清理數(shù)據(jù)。
train['license'] = train['license'].fillna('nan')
train['license'] = train['license'].astype('category').cat.codes
在一個(gè)小的自寫函數(shù)的幫助下,我對文本進(jìn)行了清理和詞形還原。函數(shù)可能很復(fù)雜,但這對于我的實(shí)驗(yàn)來說已經(jīng)足夠了。
def clean_text(text):
table = text.maketrans(
dict.fromkeys(string.punctuation))
words = word_tokenize(
text.lower().strip().translate(table))
words = [word for word in words if word not in
stopwords.words ('english')] lemmed = [WordNetLemmatizer().lemmatize(word) for word in words]
return " ".join(lemmed)
我將清理后的文本另存為新功能。
train['clean_excerpt'] = train['excerpt'].apply(clean_text)
除了文本之外,我還可以選擇 URL 中的單個(gè)單詞并將這些數(shù)據(jù)轉(zhuǎn)換為新的文本功能。
def getWordsFromURL(url):
return re.compile(r'[\:/?=\-&.]+',re.UNICODE).split(url)
train['url_legal'] = train['url_legal'].fillna("nan").apply(getWordsFromURL).apply(
lambda x: " ".join(x))
我從文本中創(chuàng)建了幾個(gè)新特征——這些是各種統(tǒng)計(jì)信息。同樣,有很大的創(chuàng)造力空間,但這些數(shù)據(jù)對我們來說已經(jīng)足夠了。這些功能的主要目的是對基線模型有用。
def get_sentence_lengths(text):
tokened = sent_tokenize(text) lengths
= []
for idx,i in enumerate(tokened):
splited = list(i.split(" "))
lengths.append(len(splited))
return (max (長度),
min(lengths),
round(mean(lengths), 3))
def create_features(df):
df_f = pd.DataFrame(index=df.index)
df_f['text_len'] = df['excerpt'].apply(len)
df_f['text_clean_len']= df['clean_excerpt']。 apply(len)
df_f['text_len_div'] = df_f['text_clean_len'] / df_f['text_len']
df_f['text_word_count'] = df['clean_excerpt'].apply(
lambda x : len(x.split(') ')))
df_f[['max_len_sent','min_len_sent','avg_len_sent']] = \
df_f.apply(
lambda x: get_sentence_lengths(x['excerpt']),
axis=1, result_type='expand')
return df_f
train = pd.concat(
[train, create_features(train)], axis=1, copy=False, sort=False)
basic_f_columns = [
'text_len'、'text_clean_len'、'text_len_div'、'text_word_count'、
'max_len_sent'、'min_len_sent'、'avg_len_sent']
當(dāng)數(shù)據(jù)稀缺時(shí),很難檢驗(yàn)假設(shè),結(jié)果通常也不穩(wěn)定。因此,為了對結(jié)果更有信心,我更喜歡在這種情況下使用 OOF(Out-of-Fold)預(yù)測。
基線
我選擇Catboost作為模型的免費(fèi)庫。Catboost 是一個(gè)高性能的開源庫,用于決策樹上的梯度提升。從 0.19.1 版開始,它支持開箱即用的 GPU 分類文本功能。主要優(yōu)點(diǎn)是 CatBoost 可以在您的數(shù)據(jù)中包含分類函數(shù)和文本函數(shù),而無需額外的預(yù)處理。
在非常規(guī)情緒分析:BERT 與 Catboost 中,我擴(kuò)展了 Catboost 如何處理文本并將其與 BERT 進(jìn)行了比較。
這個(gè)庫有一個(gè)殺手锏:它知道如何使用嵌入。不幸的是,目前,文檔中對此一無所知,很少有人知道 Catboost 的這個(gè)優(yōu)勢。
!pip install catboost
使用 Catboost 時(shí),我建議使用 Pool。它是一個(gè)方便的包裝器,結(jié)合了特征、標(biāo)簽和進(jìn)一步的元數(shù)據(jù),如分類和文本特征。
為了比較實(shí)驗(yàn),我創(chuàng)建了一個(gè)僅使用數(shù)值和分類特征的基線模型。
我寫了一個(gè)函數(shù)來初始化和訓(xùn)練模型。順便說一下,我沒有選擇最佳參數(shù)。
def fit_model_classifier(train_pool, test_pool, **kwargs):
model = CatBoostClassifier(
task_type='GPU',
iterations=5000,
eval_metric='AUC',
od_type='Iter',
od_wait=500,
l2_leaf_reg=10,
bootstrap_type='Bernoulli ',
subsample=0.7,
**kwargs
)
return model.fit(
train_pool,
eval_set=test_pool,
verbose=100,
plot=False,
use_best_model=True)
對于OOF的實(shí)現(xiàn),我寫了一個(gè)小而簡單的函數(shù)。
def get_oof_classifier(
n_folds, x_train, y, embedding_features,
cat_features, text_features, tpo, seeds,
num_bins, emb=None, tolist=True):
ntrain = x_train.shape[0]
oof_train = np.zeros((len(seeds), ntrain, num_bins))
models = {}
for iseed, seed in enumerate(seeds):
kf = StratifiedKFold(
n_splits=n_folds,
shuffle=True,
random_state=seed)
for i, (tr_i, t_i) in enumerate(kf.split(x_train, y)):
if emb and len(emb) > 0:
x_tr = pd.concat(
[x_train.iloc[tr_i, :],
get_embeddings(
x_train.iloc[tr_i, :], emb, tolist)],
axis=1, copy=False, sort=False)
x_te = pd.concat(
[x_train.iloc[t_i, :],
get_embeddings(
x_train.iloc[t_i, :], emb, tolist)],
axis=1, copy=False, sort=False)
columns = [
x for x in x_tr if (x not in ['excerpt'])]
if not embedding_features:
for c in emb:
columns.remove(c)
else:
x_tr = x_train.iloc[tr_i, :]
x_te = x_train.iloc[t_i, :]
columns = [
x for x in x_tr if (x not in ['excerpt'])]
x_tr = x_tr[columns]
x_te = x_te[columns]
y_tr = y[tr_i]
y_te = y[t_i]
train_pool = Pool(
data=x_tr,
label=y_tr,
cat_features=cat_features,
embedding_features=embedding_features,
text_features=text_features)
valid_pool = Pool(
data=x_te,
label=y_te,
cat_features=cat_features,
embedding_features=embedding_features,
text_features=text_features)
model = fit_model_classifier(
train_pool, valid_pool,
random_seed=seed,
text_processing=tpo
)
oof_train[iseed, t_i, :] = \
model.predict_proba(valid_pool)
models[(seed, i)] = model
oof_train = oof_train.mean(axis=0)
return oof_train, models
我將在下面寫關(guān)于get_embeddings函數(shù),但它現(xiàn)在不用于獲取模型的基線。
我使用以下參數(shù)訓(xùn)練了基線模型:
columns = ['license', 'url_legal'] + basic_f_columns
oof_train_cb, models_cb = get_oof_classifier(
n_folds=5,
x_train=train[columns],
y=train['target_q'].values,
embedding_features=None,
cat_features=['license'],
text_features=['url_legal'],
tpo=tpo,
seeds=[0, 42, 888],
num_bins=num_bins
)
訓(xùn)練模型的質(zhì)量:
roc_auc_score(train['target_q'], oof_train_cb, multi_class="ovo")
AUC:0.684407
現(xiàn)在我有了模型質(zhì)量的基準(zhǔn)。從數(shù)字來看,這個(gè)模型很弱,我不會(huì)在生產(chǎn)中實(shí)現(xiàn)它。
嵌入
您可以將多維向量轉(zhuǎn)換為嵌入,這是一個(gè)相對低維的空間。因此,嵌入簡化了大型輸入的機(jī)器學(xué)習(xí),例如表示單詞的稀疏向量。理想情況下,嵌入通過在嵌入空間中將語義相似的輸入彼此靠近放置來捕獲一些輸入語義。
有很多方法可以獲得這樣的向量,我在本文中不考慮它們,因?yàn)檫@不是研究的目的。但是,以任何方式獲得嵌入對我來說就足夠了;最重要的是他們保存了必要的信息。在大多數(shù)情況下,我使用目前流行的方法——預(yù)訓(xùn)練的 Transformer。
from sentence_transformers import SentenceTransformer
STRANSFORMERS = {
'sentence-transformers/paraphrase-mpnet-base-v2': ('mpnet', 768),
'sentence-transformers/bert-base-wikipedia-sections-mean-tokens': ('wikipedia', 768)
}
def get_encode(df, encoder, name):
device = torch.device(
"cuda:0" if torch.cuda.is_available() else "cpu")
model = SentenceTransformer(
encoder,
cache_folder=f'./hf_{name} /'
)
model.to(device)
model.eval()
return np.array(model.encode(df['excerpt']))
def get_embeddings(df, emb=None, tolist=True):
ret = pd.DataFrame(index=df.index)
for e, s in STRANSFORMERS.items():
if emb and s[0] not in emb:
continue
ret[s[0]] = list(get_encode(df, e, s[0]))
if tolist:
ret = pd.concat(
[ret, pd.DataFrame(
ret[s[0]].tolist(),
columns=[f'{s[0]}_{x}' for x in range(s[1])],
index=ret.index)],
axis=1, copy=False, sort=False)
return ret
現(xiàn)在我有了開始測試不同版本模型的一切。
楷模
我有幾種擬合模型的選項(xiàng):
- 文字特征;
- 嵌入特征;
- 嵌入特征,如分離的數(shù)字特征列表。
我一直在訓(xùn)練這些選項(xiàng)的各種組合,這使我能夠得出嵌入可能有多有用的結(jié)論,或者,這可能只是一種過度設(shè)計(jì)。
例如,我給出了一個(gè)使用所有三個(gè)選項(xiàng)的代碼:
columns = ['license', 'url_legal', 'clean_excerpt', 'excerpt']
oof_train_cb, models_cb = get_oof_classifier(
n_folds=FOLDS,
x_train=train[columns],
y=train['target_q'].values,
embedding_features=['mpnet', 'wikipedia'],
cat_features=['license'],
text_features= ['clean_excerpt','url_legal'],
tpo=tpo, seed
=[0, 42, 888],
num_bins=num_bins,
emb=['mpnet', 'wikipedia'],
tolist=True
)
有關(guān)更多信息,我在 GPU 和 CPU 上訓(xùn)練了模型;并將結(jié)果匯??總在一張表中。
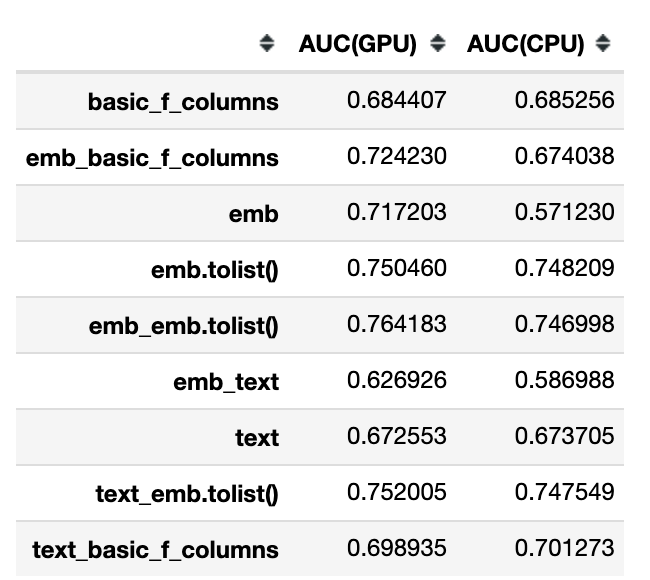
令我震驚的第一件事是文本特征和嵌入的極差交互。不幸的是,我對這個(gè)事實(shí)還沒有任何合乎邏輯的解釋——在這里,需要在其他數(shù)據(jù)集上對這個(gè)問題進(jìn)行更詳細(xì)的研究。同時(shí),請注意,將文本和嵌入用于同一文本的組合使用會(huì)降低模型的質(zhì)量。
對我來說另一個(gè)啟示是在 CPU 上訓(xùn)練模式時(shí)嵌入不起作用。
現(xiàn)在是一件好事——如果你有一個(gè) GPU 并且可以獲得嵌入,那么最好的質(zhì)量是當(dāng)你同時(shí)使用嵌入作為一個(gè)特征和一個(gè)單獨(dú)的數(shù)字特征列表時(shí)。
總結(jié)
在這篇文章中,我:
- 選擇了一個(gè)小的免費(fèi)數(shù)據(jù)集進(jìn)行測試;
- 為文本數(shù)據(jù)創(chuàng)建了幾個(gè)統(tǒng)計(jì)特征,以使用它們來創(chuàng)建基線模型;
- 測試了嵌入、文本和簡單特征的各種組合;
- 得到了一些不明顯的見解。